Email Spam Filtering Using Machine Learning: A Game-Changer for Businesses
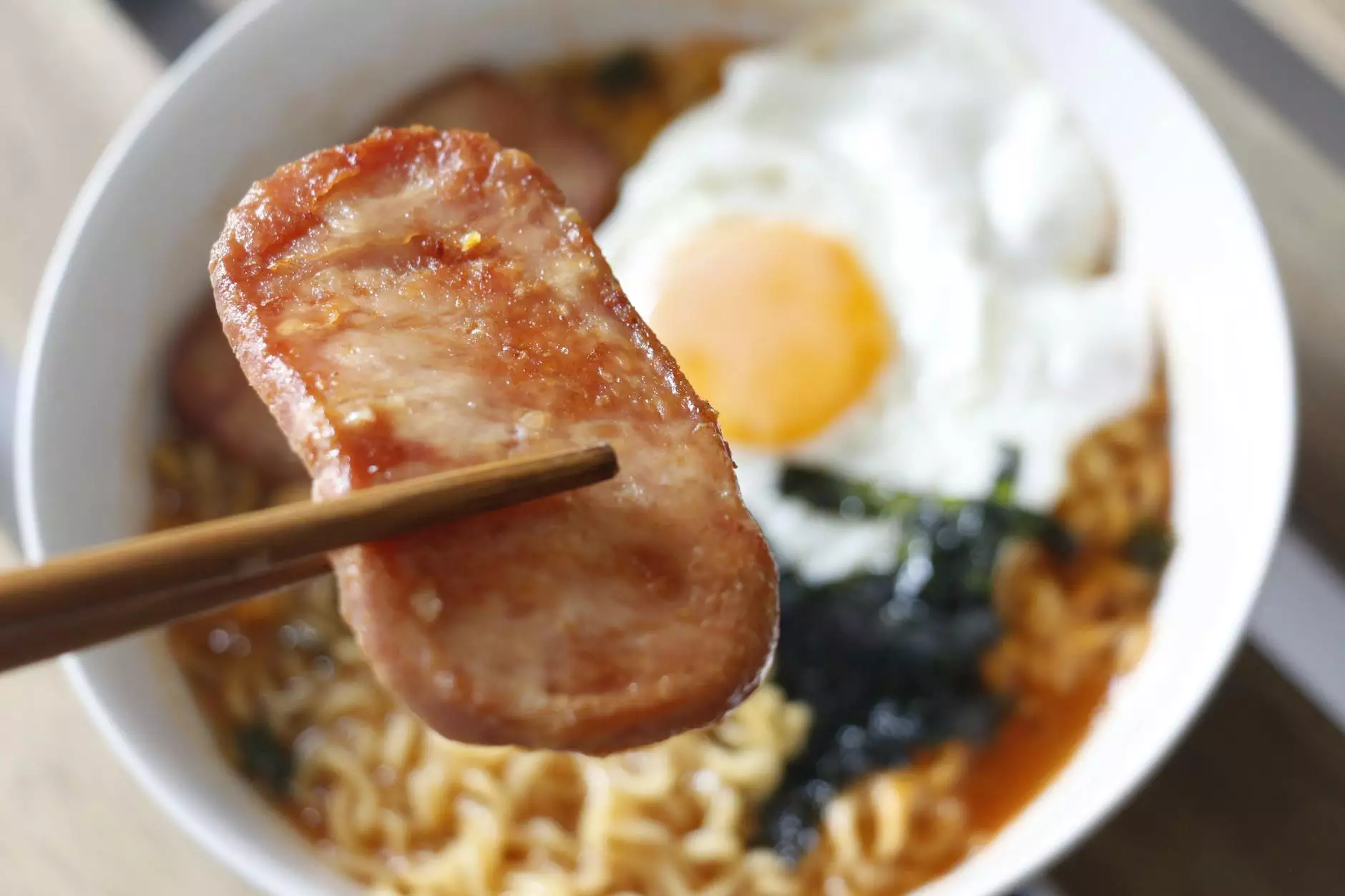
In the rapidly evolving world of technology, email communication remains a cornerstone for businesses of all sizes. However, the surge in spam emails poses a significant threat, cluttering inboxes, wasting resources, and jeopardizing sensitive information. Organizations need to adopt innovative solutions to combat this challenge, and email spam filtering using machine learning stands at the forefront of these advancements. This article delves into the intricacies of email spam filtering, its importance for businesses, and the transformative potential of machine learning techniques.
Understanding the Importance of Email Spam Filtering
Effective email spam filtering is critical for several reasons:
- Improved Productivity: By filtering out irrelevant and malicious emails, employees can focus on what truly matters, reducing distractions and enhancing productivity.
- Enhanced Security: Spam emails are often vehicles for phishing attempts and malware. A robust spam filtering system protects your business from these security breaches.
- Cost Efficiency: Reducing the volume of spam can lead to lower bandwidth usage and decrease in storage requirements, saving organizations significant costs.
- Preserved Reputation: Managing spam effectively helps maintain a professional image by ensuring that only legitimate communications reach your stakeholders.
The Challenges of Traditional Spam Filters
Traditional email spam filters typically rely on rule-based approaches that match emails against known spam signatures or patterns. While these methods can be effective, they have inherent limitations:
- Static Nature: Traditional filters cannot adapt to new spam techniques as quickly as they emerge.
- False Positives: Valuable emails may be incorrectly classified as spam, leading to potential loss of important communications.
- Limited Contextual Understanding: Basic filters often fail to grasp the subtle cues that indicate whether an email is spam or not.
Machine Learning: The Next Frontier in Email Spam Filtering
To address these challenges, businesses are increasingly turning to machine learning. Unlike traditional methods, machine learning algorithms can analyze vast amounts of data, detect patterns, and make informed predictions. Here’s how machine learning transforms email spam filtering:
1. Dynamic Learning Capabilities
Machine learning models continuously learn and adapt from new data. They leverage historical email data to update their algorithms, thus improving accuracy in identifying spam emails over time. This dynamic learning is crucial, as spammers often change tactics to evade detection.
2. Enhanced Pattern Recognition
With machine learning, filters become adept at identifying subtle patterns in email content, headers, and even user behavior. Algorithms can discern between harmless promotional emails and more malicious attempts based on contextual analysis, leading to fewer false positives.
3. Behavioral Analysis
Machine learning enables businesses to analyze user behavior and preferences. By understanding the typical email interaction patterns of users, the system can better predict which incoming emails may be spam. This personalized filtering approach enhances accuracy and user satisfaction.
4. Real-Time Processing
Machine learning algorithms can analyze emails in real-time, quickly determining whether an email should be marked as spam. This rapid processing minimizes delays and ensures that legitimate emails reach the inbox promptly.
Implementing Machine Learning for Email Spam Filtering
Implementing machine learning for email spam filtering requires a structured approach. Here are key steps that businesses should consider:
1. Data Collection
The first step involves gathering a substantial dataset of emails, including both spam and legitimate emails. The diversity in this dataset is essential for training an effective model. Consider:
- Gathering historical email data from your organization.
- Incorporating publicly available datasets for broader context.
2. Feature Selection
Next, identify the features that the machine learning model will use to classify emails. Features may include:
- Email subject lines
- Sender information
- Email content and the presence of specific keywords
- Links and attachments
- User interaction history
3. Model Training
Utilize algorithms such as Naive Bayes, Support Vector Machines (SVM), or Neural Networks to train your spam filter. The model should learn from the features of the collected dataset to distinguish between spam and legitimate emails.
4. Evaluation and Tuning
Once the model is trained, it’s crucial to evaluate its performance. Use metrics like:
- Accuracy: The ratio of correctly classified emails to the total emails.
- Precision: The ratio of true positive results to the total predicted positives.
- Recall: The ratio of true positive results to the total actual positives.
Based on these metrics, refine and tune your model for improved performance.
5. Continuous Monitoring and Updating
Machine learning models are not 'set and forget' solutions. Continuous monitoring of the model's performance is essential to ensure it adapts to evolving spam tactics. Regularly update the dataset and retrain the model to maintain its effectiveness.
Benefits of Email Spam Filtering Using Machine Learning
Adopting email spam filtering through machine learning offers significant benefits for businesses:
1. Improved Email Safety
By effectively filtering out spam, businesses enhance their email security. This protection against phishing attacks and malware helps safeguard sensitive company and customer data.
2. Increased Efficiency
With less spam in inboxes, employees can focus on critical business operations, leading to increased efficiency and productivity. This focus translates into better employee satisfaction and morale.
3. Cost-Effectiveness
Reducing spam translates to lower costs associated with data storage and bandwidth. Additionally, diminishing the risk of data breaches can save organizations from potential legal and financial liabilities.
4. Enhanced User Experience
A tailored email filtering experience that learns user preferences leads to a more personalized approach. This type of refinement builds user trust and confidence in the email system.
The Future of Email Spam Filtering
As advancements in machine learning and artificial intelligence continue to evolve, the landscape of email spam filtering will undoubtedly transform. Future trends to anticipate include:
- Integration with AI: More sophisticated AI models will emerge, enabling deeper contextual understanding and more nuanced spam detection.
- Greater User Customization: Users will have more control over their spam filters, allowing them to set specific parameters for what they consider spam.
- Collaboration Across Platforms: Cross-platform integration may allow spam filtering systems to share intelligence and updates, enhancing overall defense mechanisms against spam.
Conclusion
Email spam filtering using machine learning is not just a technological advancement; it represents a fundamental shift in how businesses manage digital communication. With the increasing volume of spam and cyber threats, implementing machine learning solutions is essential for enhancing security, improving productivity, and fostering a secure working environment. By proactively adopting these strategies, businesses can ensure they stay ahead of malicious threats while allowing their employees to focus on growth and innovation. Organizations like Spambrella.com are leading the way in providing innovative IT services and security systems tailored to meet these needs, making them a valuable partner in navigating the complexities of email communication.